Stable Diffusion is a text-to-image machine learning model developed by Stability AI, which has quickly gained popularity among people looking to create great art by simply describing their ideas through words. The Stable Diffusion image generator is based on a type of diffusion model called Latent Diffusion. Stability AI also uses various sampling types when generating images. Learn more about the different types and the best stable diffusion sampler in this article.
The basics of stable diffusion samplers
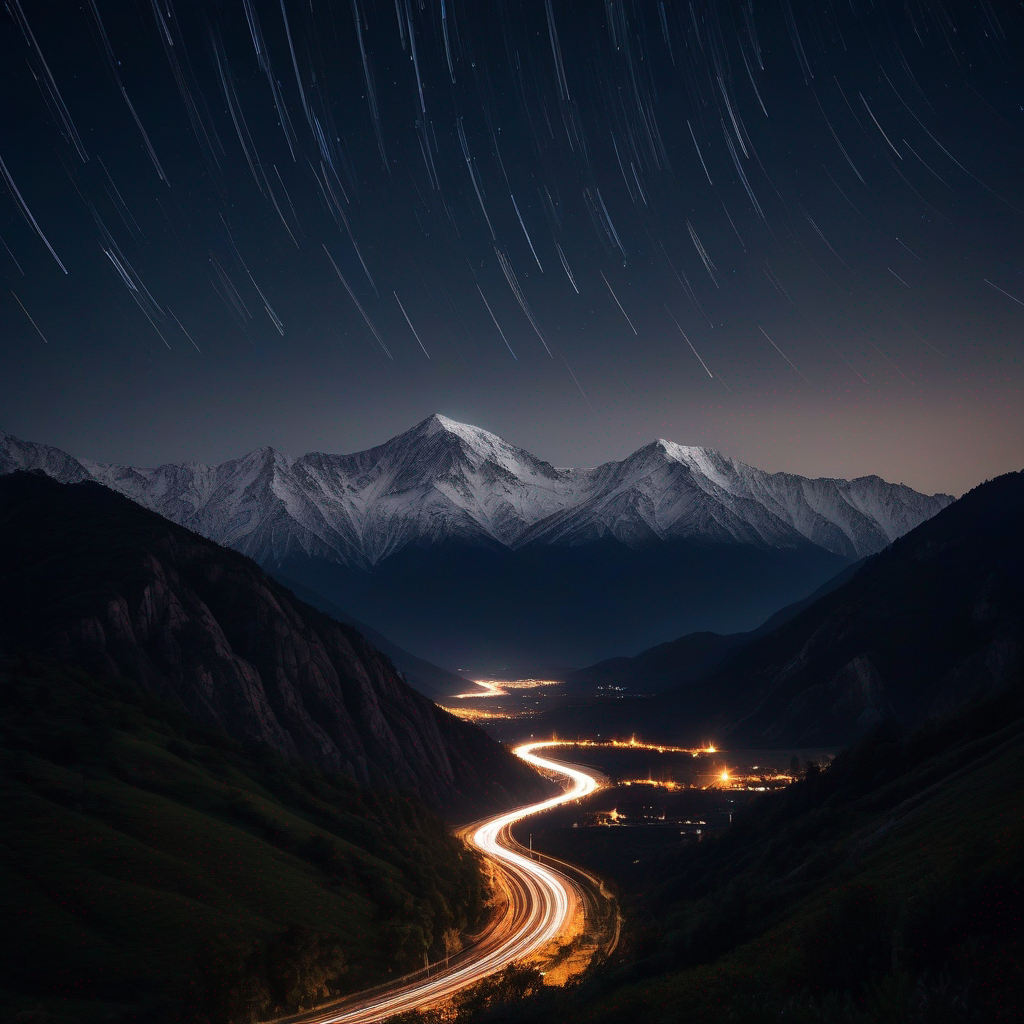
Diffusion models, in general, are machine learning algorithms trained to gradually remove undesirable noise from a resulting sample. Different prompts or descriptions must be separated by commas to allow the model to know the different parameters that help define your image. To create an image, you must first have a clear idea of what you’re looking for. Stable Diffusion uses a term, phrase, or a group of words and phrases to build an ideal image. The more details you provide, the higher the likelihood you will get the desired outcome. The system uses trial and error to fine-tune your prompts.
Variational Autoencoder (VAE) in Stable Diffusion Samplers:
Stability AI uses various sampling types when generating images. In general, samples allow for the development of finer details using a variational autoencoder (VAE), a type of artificial neural network that is used for unsupervised learning of complex distributions. VAE is a generative model that is trained to learn a compact, lower-dimensional representation (called the latent space) of data and to generate new data samples that are similar to the training data.
VAEs are used for a variety of tasks in artificial intelligence, including image generation, natural language processing, and representation learning. They are handy for tasks that involve large and complex datasets because they can learn to extract useful features and patterns from the data in an unsupervised manner. Stability AI chose to produce images based on sampling types. Differences between samplers can be very subtle, but these parameters are highly configurable so that you can experiment with them. A lot will depend on your prompt, so feel free to try new things.
Working with diffusion models
One great benefit of diffusion models is how well they visualize data, but it has drawbacks. It can be difficult to train these models because they need precise descriptions to work effectively. Prompts need to be repeated and sequenced to create an accurate picture.
Additionally, these models can utilize a lot of computational resources, particularly when dealing with high-resolution images, so there may be instances when users encounter a CUDA out-of-memory error.
Sampling algorithms let you explore parameters efficiently, enabling quicker convergence to the desired distribution. By reducing the variance of the samples, sampling algorithms can produce estimates of the target distribution that are more precise. Sampling algorithms are also robust and can react quickly to changes in the distribution, which is helpful in circumstances where the distribution constantly evolves.
How Stable Diffusion Model works:
The stable diffusion model requires two inputs: a latent seed and a text prompt. The latent seed generates random latent image representations with dimensions of 64×64, while the text prompt is converted into text embeddings with dimensions of 77×768 using CLIP’s text encoder.
Comparing different types of Stable Diffusion samplers:
Here are some commonly used diffusion samplers (also called equilibrium samplers):
- k_LMS: The k-LMS Stable Diffusion method consists of a series of tiny, random steps that lead in the direction of the gradient of the distribution, starting from a point in the parameter space. The steps minimize sample variance by adjusting the step size based on the distribution’s curvature. It results in quicker and more effective sampling toward the target distribution.
- DDIM: The DDIM Stable Diffusion method is an extension of the k-LMS Stable Diffusion algorithm and provides more precise sampling. It further decreases sample variance and enhances convergence to the desired distribution. This is accomplished by including more details on the distribution’s curvature to the model. Unlike other algorithms, DDIM only takes eight steps to achieve incredible images.
- k_euler_a and Heun: Similar to DDIM, the k_euler_a and Heun samplers are incredibly quick and produce excellent results. However, they significantly alter the generational style. If a good image is found in k_euler and Heun, it can be moved into DDIM or the other way around until the perfect outcome is achieved.
- The k_dpm_2_a sampler: The k_dpm_2_a sampler is considered by many to be superior to others, although it trades speed for quality. It involves a thirty- to eighty-step process, but the results are incredible. It’s better suited for highly tuned prompts with minimal errors and is not the best sampler for experimentation.
When choosing the right sampler, it’s important to consider the complexity of the image, the resolution, and the type of prompt used. Each sampler has its strengths and weaknesses, and it’s up to the user to experiment and find the perfect fit for their needs.
Vroblesky (2001) recommends that the vertical distribution of contaminants be determined in wells having 10-ft-long well screens, and that both the vertical distribution of contaminants and the potential for intra-borehole flow be determined in wells having screens longer than 10 ft.
CLU-IN
Choosing the Best Stable Diffusion Sampler
Choosing the Best Stable Diffusion Sampler is crucial for ensuring accurate and reliable sampling results. By selecting a sampler that offers stability in varying environmental conditions, researchers can confidently collect data without compromising the integrity of their samples. A stable diffusion sampler not only guarantees consistent performance but also enhances the overall efficiency and effectiveness of the sampling process. It is essential to consider factors such as durability, precision, and compatibility with different sampling environments when deciding on the most suitable diffusion sampler for your research needs.
Types of Stable Diffusion Samplers:
Direct Samplers
- Concept: Derive samples directly from the distribution.
- Examples: Inversion Method, Rejection Sampling.
- Use Cases: Simple distributions, less computational cost.
Markov Chain Monte Carlo (MCMC) Samplers
- Concept: Construct a Markov chain with a desired distribution as its equilibrium.
- Examples: Metropolis-Hastings, Gibbs Sampling.
- Use Cases: Complex distributions, large-scale simulations.
Sequential Monte Carlo (SMC) Samplers
- Concept: Use sequential importance sampling with resampling steps.
- Examples: Particle Filters.
- Use Cases: Dynamic systems, state-space models.
Criteria for Choosing a Stable Diffusion Sampler:
Efficiency
- Speed: Time complexity, computational load.
- Resource Utilization: Memory, parallelization ability.
Accuracy
- Bias: Systematic errors, skewing results.
- Variance: Dispersion of sample estimates.
- Convergence: Speed and reliability of reaching equilibrium distribution.
Complexity
- Implementation: Difficulty, code availability.
- Tuning: Parameter settings, sensitivity.
Applicability
- Target Distribution: Shape, complexity.
- Dimension: Number of variables, high-dimensional spaces.
Common Applications of Stable Diffusion Samplers:
Machine Learning
- Bayesian Inference: Posterior distributions, model selection.
- Probabilistic Models: Latent variable estimation, uncertainty quantification.
Physics
- Quantum Simulations: Path integral methods, statistical averaging.
- Statistical Mechanics: Thermodynamic properties, phase transitions.
Finance
- Risk Management: Value-at-risk, stress testing.
- Option Pricing: Stochastic volatility models, future price distributions.
Stable Diffusion for free with neuroflash
Our tool for AI image creation is called ImageFlash. All neuroflash users have access to our image generation tool. We offer two quality levels – Standard and Premium. Standard images run on Stable Diffusion technology and can be generated in a wide range of aspect ratios. With our premium images, we offer AI images based on DALL-E 3. If you want to take your content to the next level, why not try the neuroflash image generator?
With neuroflash, you can quickly and easily create eye-catching visuals that will help you stand out from the competition. Plus, with the ability to optimize your image generation prompts automatically, you will have better results and save time.
Frequently Asked Questions
1. How do stable diffusion samplers work?
Stable diffusion samplers work by exploring parameters efficiently and reducing the variance of the samples, producing estimates of the target distribution that are more precise. Each sampler has its strengths and weaknesses, and the right sampler is chosen based on the complexity of the image, the resolution, and the type of prompt used.
2. What are the advantages of using stable diffusion samplers?
Stable diffusion samplers allow for the creation of incredible images based on a text prompt. By using various sampling types, they efficiently explore parameters and reduce the variance of the samples, producing precise estimates of the target distribution.
3. What are the challenges of using stable diffusion samplers?
Stable diffusion samplers require precise descriptions and sequencing to work effectively, and they can utilize a lot of computational resources, particularly when dealing with high-resolution images. This can lead to a CUDA out-of-memory error. It’s important to choose the right sampler based on the complexity of the image, the resolution, and the type of prompt used.
4. What are some tips for using stable diffusion samplers effectively?
To use stable diffusion samplers effectively, it’s important to have a clear idea of the image you want to create and provide a detailed prompt. Joining online communities for inspiration and experimenting with different samplers can also be helpful in achieving the desired outcome. For a tutorial on how to write good AI image prompts plus examples, check out this article.
5. Where can I try stable diffusion samplers?
Stable diffusion samplers are available through various AI art apps and websites.
Conclusion
In conclusion, Stable Diffusion Samplers in AI technology have opened up a new realm of possibilities for artists and designers. By using various sampling types and equilibrium samplers, stable diffusion allows users to create incredible images based on a text prompt. While each sampler has its advantages and disadvantages, it’s up to the user to experiment and find the right fit for their needs. With this technology, the